The rise of big data has revolutionized the way businesses operate in today’s world. As more and more organizations realize the potential of data-driven decision-making, the need for effective data collaboration becomes increasingly evident.
In this guide, we’ll explore the fundamentals of data collaboration for non-techies, including what it is, why it’s important, and how to implement it in your business.
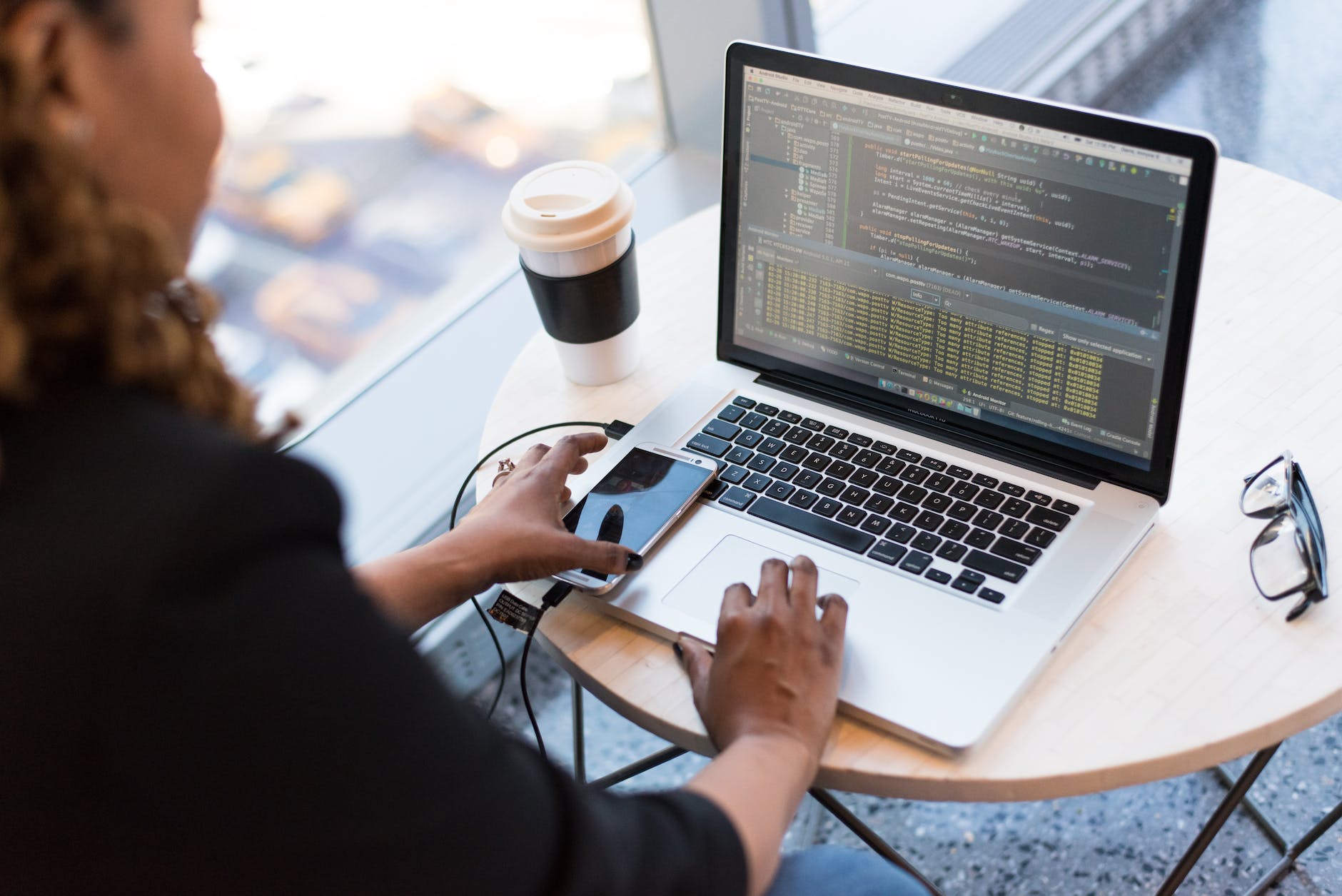
Understanding Data Collaboration
What is Data Collaboration?
Data collaboration is a process that involves sharing data and working together to analyze and make decisions based on that data. The goal of data collaboration is to gain insights that can inform strategic business decisions and improve overall performance. By utilizing shared data resources, businesses can gain valuable insights into consumer behavior, market trends, and industry best practices.
One of the key benefits of data collaboration is that it enables businesses to break down data silos. Data silos are created when data is stored in isolated systems, making it difficult to access and analyze. By integrating data from multiple sources into a single system, businesses can gain a more comprehensive understanding of their data.
The Importance of Data Collaboration in Today’s World
Data collaboration is critical for businesses looking to remain competitive in today’s rapidly evolving technological landscape. By utilizing shared data resources, businesses can gain valuable insights into consumer behavior, market trends, and industry best practices. This can improve decision-making, increase efficiency, and boost overall performance.
For example, a retail business can use data collaboration to gain insights into consumer behavior. By analyzing data from various sources, such as social media, website traffic, and sales data, the business can gain a better understanding of what products are popular and what marketing strategies are most effective.
Key Terms and Concepts in Data Collaboration
Before diving into the data collaboration process, it’s essential to have a strong grasp on some key terms and concepts in the field. Some of these include:
- Data silos: When data is stored in isolated systems, it can create silos of information that are difficult to access and analyze. Breaking down data silos is a critical component of data collaboration.
- Data integration: Combining data from multiple sources into a single system for easier analysis. Data integration is a key step in the data collaboration process.
- Data governance: The policies and procedures for managing data assets within an organization. Data governance is essential for ensuring that data is accurate, complete, and consistent.
- Data quality: The accuracy, completeness, and consistency of data. Ensuring data quality is a critical component of data collaboration.
By understanding these key terms and concepts, businesses can better prepare themselves for the data collaboration process and gain more valuable insights from their data.
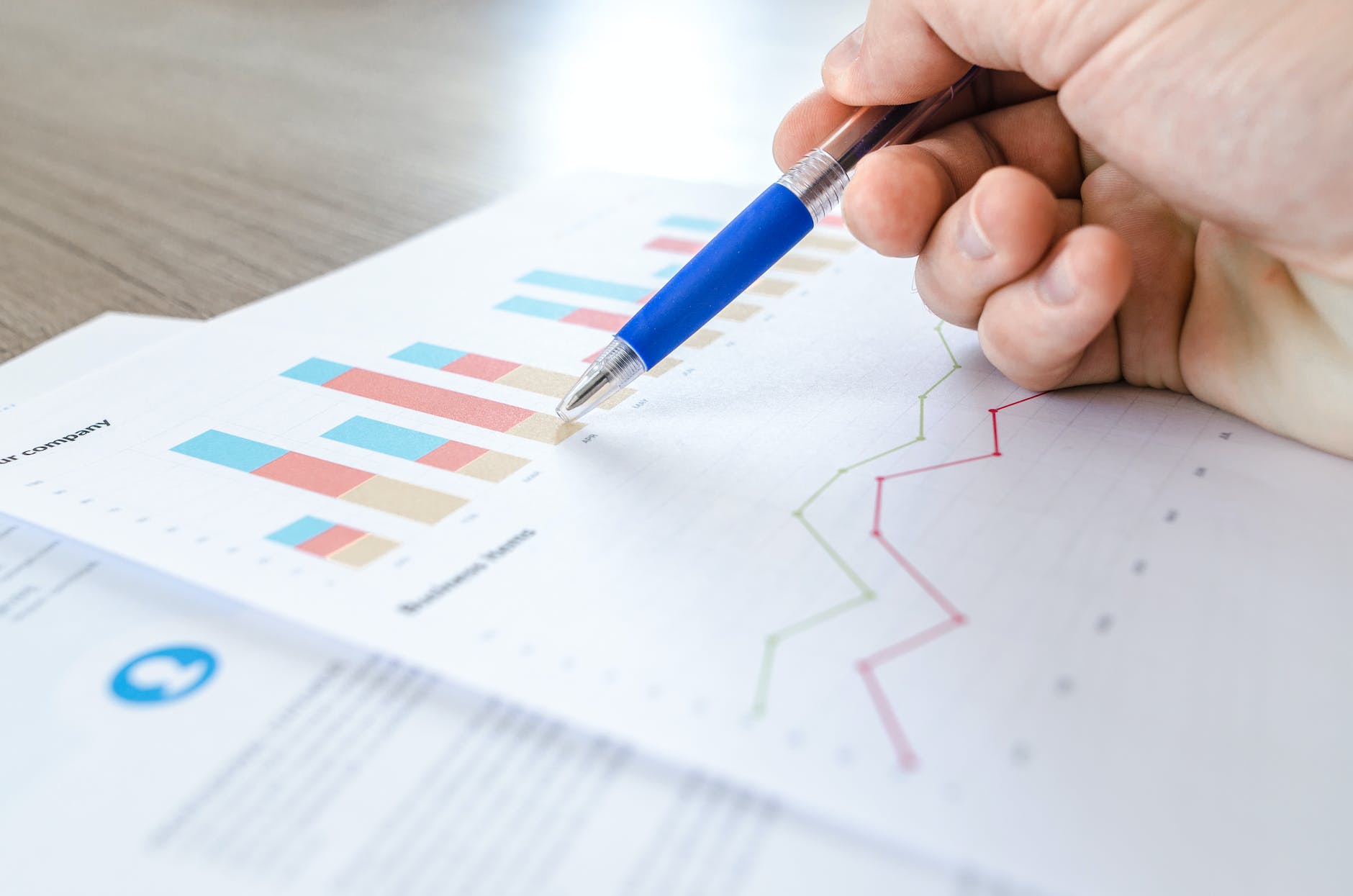
The Data Collaboration Process
Identifying Your Data Collaboration Goals
Effective data collaboration begins with identifying clear goals and objectives. This involves determining what data is most important to your business, how it will be collected, and what insights you hope to gain from it.
Assembling Your Data Collaboration Team
A successful data collaboration project requires a cross-functional team with a variety of skill sets. This might include data scientists, analysts, project managers, and subject matter experts. By bringing together a diverse group of professionals, you can ensure that all aspects of the project are covered.
Defining Roles and Responsibilities
To ensure a smooth collaboration process, it’s important to define roles and responsibilities for each team member. This includes identifying who will be responsible for data collection and analysis, as well as who will be involved in decision-making and project management.
Establishing Data Sharing Agreements
Before sharing data, it’s important to establish clear agreements regarding how it will be used and protected. This includes identifying who owns the data, how it will be accessed, and what security measures will be in place to protect it.
Tools and Platforms for Data Collaboration
Cloud-Based Data Storage Solutions
Cloud-based data storage solutions, such as AWS, Google Cloud, and Microsoft Azure, offer secure and scalable storage solutions for data collaboration projects. These platforms allow teams to store and share data seamlessly, without the need for costly hardware or complex IT infrastructure.
Data Visualization and Analysis Tools
Data visualization and analysis tools, such as Tableau, Power BI, and Google Data Studio, help teams make sense of large volumes of data by creating interactive and visually appealing reports. These tools can highlight patterns and trends that might not be immediately apparent when viewing raw data.
Communication and Project Management Platforms
Collaborative communication and project management platforms, such as Slack, Trello, and Asana, allow team members to work together in real time, regardless of their physical location. These platforms streamline communication, project tracking, and resource allocation, making data collaboration projects more efficient.
Security and Privacy Tools for Data Collaboration
Given the sensitive nature of data collaboration projects, it’s essential to have strong security and privacy measures in place. This might include encryption tools, secure data sharing platforms, and comprehensive data protection policies
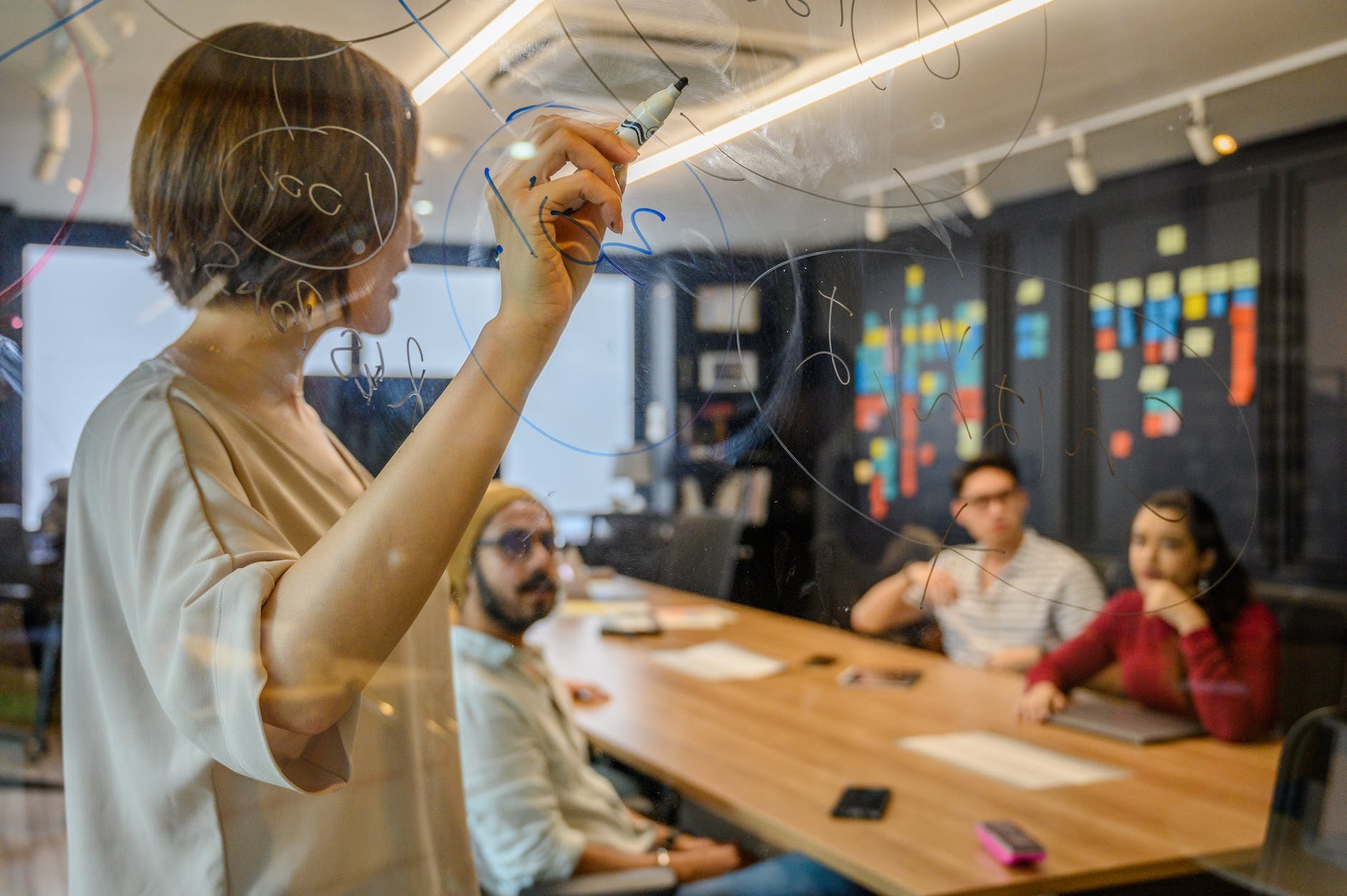
Best Practices for Effective Data Collaboration
Ensuring Data Quality and Consistency
High-quality data is essential for effective data collaboration. This means ensuring that data is accurate, complete, and consistent across all sources. Regular data audits and quality checks are important to maintain data integrity.
Establishing Clear Communication Channels
Open communication channels are critical for successful data collaboration. This includes establishing regular meetings, sharing progress reports, and maintaining ongoing communication between team members.
Implementing Version Control and Change Management
To avoid confusion and ensure that all team members are working with the most up-to-date data, it’s important to implement version control and change management protocols. This helps prevent errors and ensures that all team members are working with the same data set.
Regularly Reviewing and Updating Collaborative Processes
Data collaboration is an ongoing process that requires regular review and refinement. This includes evaluating the success of the project, identifying areas for improvement, and making necessary changes to optimize collaboration processes.
Conclusion
In conclusion, effective data collaboration requires a deep understanding of the data collaboration process, including clear goal-setting, assembling the right team, using the right tools, and implementing best practices. By following the guidelines outlined in this guide, non-techies can successfully collaborate on data-driven projects and gain valuable insights into their business operations.
TRUECHART fundamentally changes data visualization and collaboration – powered by standardized IBCS® templates. It provides the speed and scale needed to tackle petabytes of business intelligence in interactive speed to make data management and complex reporting much easier, simpler, and faster. Book your TRUECHART demo today.
Stay up-to-date with TRUECHART. Follow us on LinkedIn.